The Problem
Current BCI research tests how well a subject is able to learn a novel movement task with engineered task dynamics and limited sensory feedback compared to natural motor control. Specifically, learning to use a BCI requires the user to adapt to velocity-controlled cursor or robot arm dynamics while using only visual feedback for control. However, during natural reaching tasks, healthy subjects must provide complex control signals to overcome the inertia of the arm as well as make use of both visual and proprioceptive feedback. BCI optimization, then, requires an understanding of how the brain adapts to new task dynamics and how to enhance sensory feedback during online performance.
Our Approach
We are using a variety of BCIs to test our hypotheses regarding how the brain adapts to novel task dynamics and how to enhance online sensory feedback.
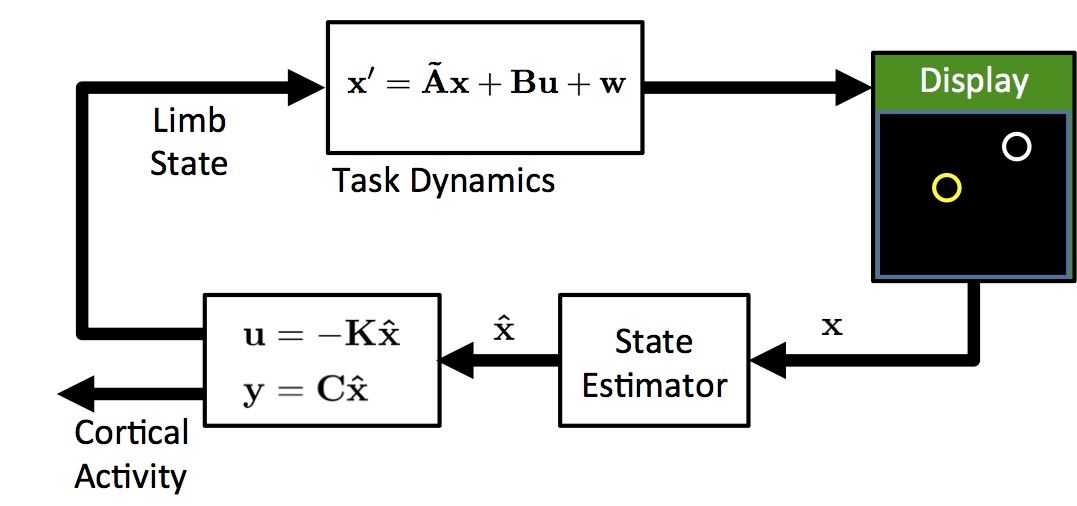
For the task dynamics focus, we are making use of data from intracortical BCI experiments performed in macaques, as well as experimentation on human subjects performing EMG-controlled tasks. Our studies range from motor cortical neural correlates of adaptation to kinematic analyses of control strategy using inverse optimal control to empirical measures of movement performance, such as Fitts’ Law.
Impact
We anticipate that a greater understanding of how users adapt to control BCIs as well as providing enhanced sensory feedback will improve rates of learning and reduce the user burden of BCI control.
Publications:
C. Matlack, A. Haddock, C. T. Moritz, H. J. Chizeck, “Motor cortical decoding performance depends on controlled system order,” the Proceedings of the 36th Annual International Conference of the IEEE Engineering in Medicine and Biology Society (EMBC), Chicago, IL, August 2014.
A. Haddock, C. Matlack, C. T. Moritz, H. J. Chizeck, “An optimal control analysis of motor strategies in a brain-computer interface task,” the Proceedings of the 6th International IEEE EMBS Neural Engineering Conference (NER), San Diego, CA, November 2013.
Abstracts:
A. Haddock, C. Matlack, H. J. Chizeck, “Neural correlates of task dynamics in motor cortex,” Society for Neuroscience 44th Annual Meeting, Washington, DC, November 2014.
A. Haddock, C. Matlack, H. J. Chizeck, “Identifying motor objectives in a brain-computer interface task by inverse optimal control,” 2013 BMES Annual Meeting, Seattle, WA, September 2014.
Funding:
Affiliated Students and Faculty: Andrew Haddock, Brady Houston, Howard Chizeck